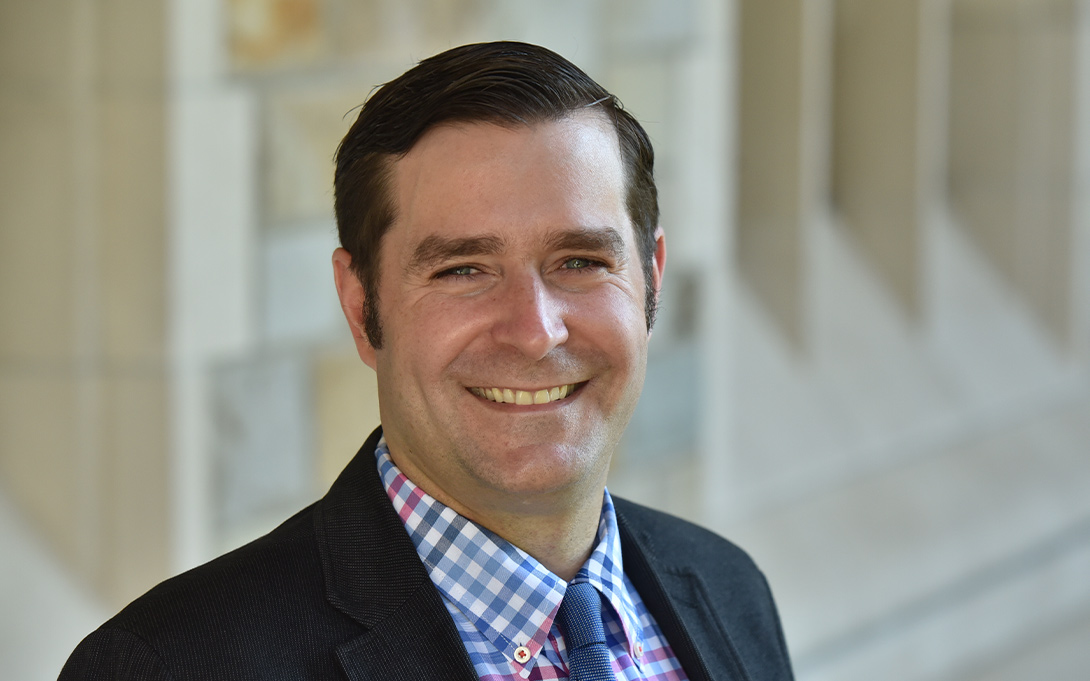
A challenge in any analysis looking at multiple higher education institutions is that each institution may have different labels for the same course - ENG101 may be "Composition I" at one institution but "Thinking Like an Engineer" at another. Ford School professor Kevin Stange wants to use various machine learning approaches to classify all courses in a systematic way using the text of course subjects, titles, and descriptions that will facilitate cross-institution analysis of the content and consequences of students' college courses. A recent award from Michigan Institute for Data Science (MIDAS) will help him in his efforts.
Stange is co-leading the College and Beyond II: Outcomes of a Liberal Arts Education project, with Ford School professor Paul Courant among others a 3½ year initiative at U-M’s Inter-university Consortium for Political and Social Research (ICPSR). The machine learning algorithm will aim to classify the content of more than 50 million courses taken by two million students from multiple institutions into an existing hierarchical taxonomy called the College Course Map (CCM). The widespread adoption of a standard course content system (whether CCM or others) has been hampered by two daunting tasks: the sheer scale of human intervention typically required to manually classify thousands of unique courses within an institution and a lack of standardization of course subjects and numbering across multiple institutions.
The MIDAS award, a Propelling Original Data Science (PODS) grant, “strongly encourages works that transform research domains through data science and AI, works that improve the reproducibility of research, and works that promise major impact and potential for significant expansion.”
“We are thrilled by the many brilliant research ideas in the large number of submitted proposals and wish that we could fund many more. The diverse range of research that MIDAS is able to fund demonstrates the strength of data science and AI research at U-M,” says Dr. H. V. Jagadish, MIDAS Director.
Stange, who is also faculty co-director of the Education Policy Initiative, says, “This project represents a transformative use of data science in the domain of educational scholarship and practice by making CCM classification possible on a previously unimagined scale.”
“The MIDAS funding will also facilitate our application for major external grant funding to hopefully scale up the classification approach to include additional features, test it on additional applications, and maybe even develop an open-source software package that will be made widely available through ICPSR to be used by postsecondary institutions and researchers,” he adds.